
Autonomous experimentation is an emerging area of research that has now been extended to infrared (IR) spatiochemical mapping of dynamic biological systems. Such approaches have been difficult to apply to these traditionally high-dimensional mapping technologies, which include scanning hyperspectral imaging of biological systems, due to the inherent complexity and heterogeneity of typical samples. In this review, researchers present the history of adaptive sampling algorithms and surrogate modeling and summarize the recent implementations of autonomous adaptive data acquisition (AADA) for scanning hyperspectral imaging.
IR hyperspectral imaging of biological systems is a non-invasive and label-free method for studying the time-resolved spatiochemical evolution of living biological systems. Using traditional approaches, staff scientists supporting user research activities at synchrotron facilities devote a significant amount of time to identifying regions of interest in user samples, which limits the efficiency of beamline and the number of users able to access such facilities. With advances in AADA, the time required to complete a user’s full data collection protocol can be significantly reduced by employing the system’s ability to autonomously and adaptively locate and analyze sample features of interest, thereby enhancing overall efficiency and increasing the number of users that can be served.
Advances in autonomous experimentation, which is closely linked to the original concepts of artificial intelligence, have traditionally been limited by two recurring primary challenges: distribution shifts due to discrepancies between training data sets and the conditions during testing or deployment, and difficulties in transfer learning, in which information gleaned from a previously learned task must be transferred to a new task. While many potential solutions are possible, the authors state that the simplest is to separate the autonomous experimentation workflow into distinct, actionable components that can individually be user-optimized for a given user’s specific structural and functional goals.
In this sense, the authors of this review conceptualize AADA as a flexible, tunable, “smart” building block framework that can be embedded into scanning hyperspectral imaging workflows to obtain high resolution and high precision chemical information of samples. The authors also present multiple example use scenarios to illustrate this concept.
Funding acknowledgements
This work was supported by the Howard Hughes Medical Institute (HHMI) under Grant No. 047-101, and the U.S. Department of Energy, Office of Science, Office of Biological and Environmental Research under Contract No. DEAC02-05CH11231.
Related links
- Scilight features overview of the study, by AIP Publishing
References
- Holman EA, Krishnan H, Holman DR, Holman HYN, and Sternberg PW. “Toward implementing autonomous adaptive data acquisition for scanning hyperspectral imaging of biological systems”, Appl. Phys. Rev. 10: 011319. (2023; https://aip.scitation.org/doi/pdf/10.1063/5.0123278)
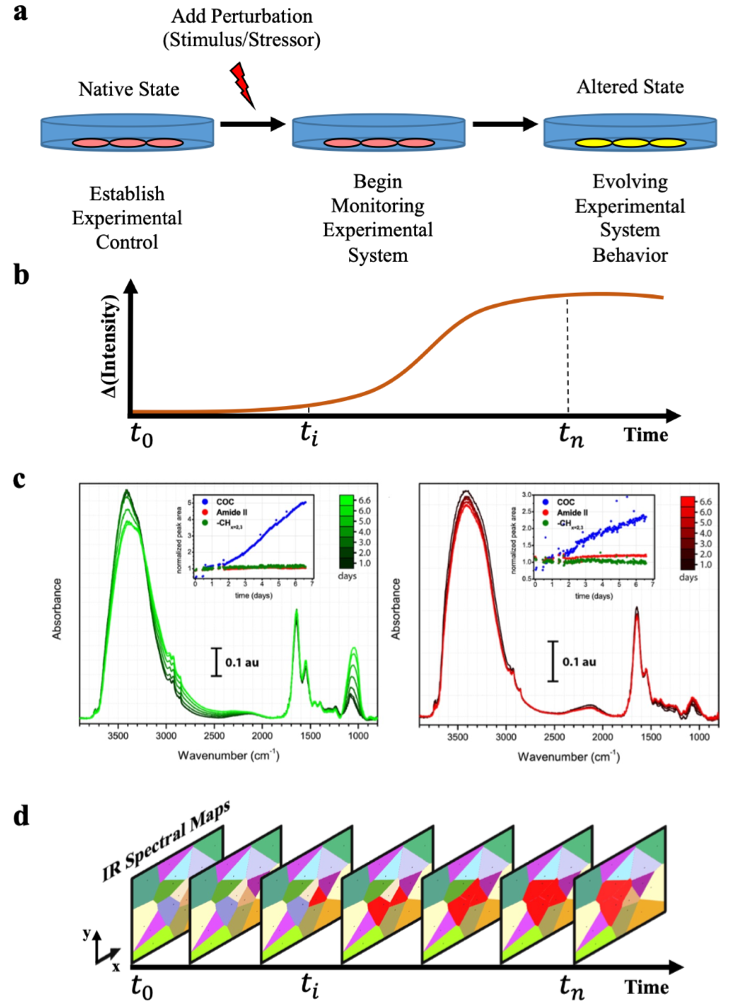
Schematic of a generalized living biological system study to develop autonomous adaptive data acquisition (AADA) for time-resolved scanning hyperspectral imaging of dynamic systems. (a) Depiction of a general experimental design that monitors a system for an unknown behavioral response to a stimulus. (b) Simplified depiction of the spectral change over time of an otherwise high-dimensional space of broadband IR spectra. (c) Reproduced with permission from Anal. Chem. 87, 9 (2015). Copyright 2015 American Chemical Society. Multiday IR spectral progression measurements of PC12 cell colonies showing spatiochemical and spatiotemporal differences between cells. (d) Concept of temporally resolved spatiochemical mapping corresponding to the system’s evolution timeframe in (a) and (b). [Reprinted with permission from the authors.]
By Liz Boatman